What’s the relationship between IoT and big data?
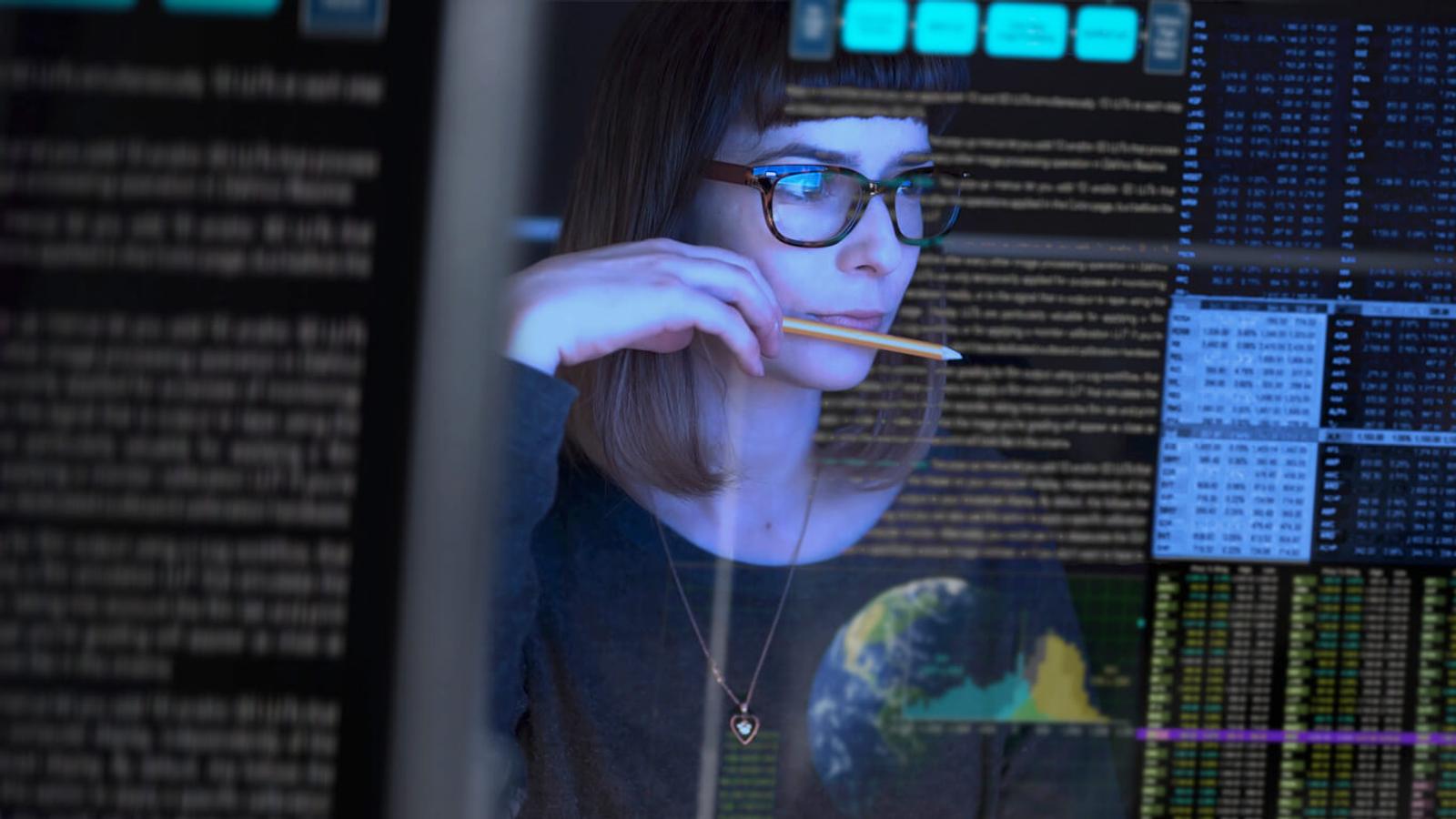
IoT sensors and devices generate tremendous amounts of data, and businesses are using analytics to interpret it.
The idea of big data has its roots in the 1960s when computers began to store and process large data sets in data centers. However, the term big data didn’t really emerge until the early 2000s, when statisticians realized the magnitude of information collected online—especially via social media and e-commerce platforms. New software tools such as Hadoop enabled big data analytics on a new level and paved the way for distributed data storage.
While human interaction with online platforms painted the early picture of big data (for example, Amazon’s product recommendation algorithm is based on data it collects from individual users), the internet of things (IoT) is increasingly driving its development. IoT sensors and devices generate tremendous amounts of data, and businesses are using analytics to interpret it.
Use the links below to jump to what you need:
How does IoT impact big data (and vice versa)?
How can IoT and big data benefit industries?
The future of IoT and big data
Connectivity
Billions of connected devices, sensors, and other “things” now collect more data than the world has ever seen. This ubiquitous level of connectivity, powered in part by cellular technologies, results in enormous data sets that organizations must sift through and analyze if they want to derive value. Powerful processors and software tools are needed to deal with such massive collections of information. That’s where the term “big data” comes in.
What is big data?
Big data is exactly what it sounds like: tremendous data sets that cannot be processed without modern computing technology. Big data is rife with hidden insights — for example, consumer trends that are only detectable if you review millions upon millions of business transactions. Today’s cloud computing processors can do that level of work, making it possible to harness the power of big data to deliver insights for businesses, healthcare organizations, governments, and researchers in many sectors.
To define the term, IBM originated the “Three Vs” of big data. More recently, the list has expanded to five:
Volume
Big data typically involves large quantities (terabytes or petabytes) of chaotic, unstructured information. To utilize the data, organizations must find ways to sort and manage it.
Velocity
Big data isn’t only high in volume — it’s also streaming in at a high velocity (imagine a fire hose). In a digitally connected world, data is generated at staggering speeds, and organizations must find ways to keep up — especially if they need to extract real-time insights.
Variety
Big data is quite varied in format and can include numerical data, text, video, or audio information. And an organization’s data might come from many different sources — social media, customer reviews, enterprise systems, and IoT sensors — making it difficult to consolidate.
Value
Big data has intrinsic value, yet that value must be extracted. Discovering the value in an organization’s data set requires powerful analytical tools, intelligent data scientists, and executives who are open to hearing what the data has to say. Predictive analytics is one area that can yield great value, as it helps leaders make predictions based on trends they discern in the data.
Veracity
Facts don’t lie, but in its complexity, big data can be deceiving. Data scientists must be careful to cleanse data of errors, duplicate information, and other anomalies that can disrupt their ability to find true insights.
As the field of big data analytics has unfolded, so too have sophisticated methods for uncovering insights.
AI and machine learning
Artificial Intelligence (AI)
AI is a broad term that generally refers to making computers behave in ways that mimic human intelligence. But the parameters of that description are unclear and always changing. For example, the ability to play a game of chess was considered a form of AI several decades ago. Today, nearly every computer’s operating system has that capability, and typical examples of AI are much more complex (like self-driving cars). Most computer scientists use AI as an umbrella term under which several other technologies fall: machine learning, deep learning, IoT sensors, computer vision, and autonomous robots, among others.
Machine Learning (ML)
A subset of AI, ML “is the study of computer algorithms that allow computer programs to automatically improve through experience,” according to computer scientist Tom M. Mitchell. Closely related to data analytics, ML looks at datasets to identify patterns and “learn” from recurring events. One common example of ML is the recommendation algorithms used by many movie and music streaming services. Once the system knows what kind of entertainment you enjoy, it can suggest other songs or movies that you might like.
A subcategory of ML is deep learning, also known as deep structured learning. It uses neural networks without the domain-specific feature engineering required by other types of machine learning. The “deep” qualifier refers to multiple layers of neural networks, which are often used in applications such as computer vision and chatbots.
How does IoT impact big data (and vice versa)?
A fast-growing network of connected sensors around the world, the internet of things is making a colossal contribution to the body of big data. Here are three ways it impacts the field of big data:
Generates a variety of data
As connected devices move beyond the realm of consumer electronics, a wide variety of different kinds of data come into play. IoT sensors can collect information about water levels in farm fields, seismic conditions underground, vital signs in remote patient monitoring, and much more. Having different types of data in a specific use case helps analysts get a more accurate picture of what’s going on and yields deeper insights. For example, in a smart factory, combining data from human workers and connected machines — and from enterprise systems and the factory floor (bridging the OT/IT divide) — results in greater efficiency and productivity.
Increases data volume
Along with providing new and different types of data, IoT sensors also deliver incredible data volume. Companies implementing an IoT device deployment often don’t anticipate the tsunami of data that will come their way once the devices are switched on. To make the most of it, they will need systems in place to store and process it, preferably in real-time (depending on the use case). This is why the proliferation of IoT devices is driving an increasing need for high-speed edge processors and flexible, cloud-based storage systems.
Enables real-time analytics
Because it depends on machines to gather data, rather than humans, IoT creates data sets very quickly — often in real-time. Achieving real-time analytics depends on machine-driven tools like AI, machine learning, and deep learning. The insights they uncover can then make an impact on IoT devices, users, and organizational decisions.
Here’s an example: traffic lights and traffic cameras are connected to a smart city’s IoT network. One camera reveals regular traffic jams at an interchange around rush hour each day, causing traffic to back up onto the interstate. AI tools analyze the data and suggest a solution: increasing the frequency and duration of green lights for traffic exiting the interstate during that period of time each day. Since the traffic lights are also connected to the network, city leaders can easily make that adjustment and cause traffic to flow more smoothly during peak hours.
How can IoT and big data benefit industries?
The combination of IoT and big data analytics can empower organizations in many industries to achieve greater efficiencies, anticipate potential problems before they arise, and make smarter decisions. Analytics reveals patterns and trends in data, uncovering insights that enable predictive maintenance for machines and help businesses better understand their customers. Here are a few examples of how the marriage of IoT and big data can impact various industries:
Healthcare
As telehealth and telemedicine continue to expand, connected health monitors will become common components of the healthcare system. These devices enable providers to track patients’ readings remotely — from connected blood pressure meters to glucometers and heart monitors, just to name a few. As the connected sensors collect and aggregate data, AI and machine learning tools can identify trends and warning signs, alert patients and providers, and potentially save lives.
Another application of big data and IoT is healthcare data management. IoT tracking systems can help hospitals monitor the location of equipment and people. Data portals and management systems allow providers to aggregate patient information, including electronic health records (EHRs) — improving access and helping to reduce human error. Applications of healthcare analytics can provide predictive recommendations for patient care, hospital management, and more.
Supply chain management
Supply chain applications of IoT include asset trackers, telematics systems, and remote monitoring systems. These devices generate tremendous amounts of disparate data that can be difficult to combine and interpret — but AI and machine learning are helping.
For example, a shipping company might have a thousand trucks traversing the country’s interstates at a given moment. Inside the truck, containers are equipped with asset trackers, while the vehicle itself has an onboard telematics system. Using a centralized cloud platform, the company can view all this information on a single dashboard, along with similar data from the rest of the fleet. AI and machine learning tools can identify trends and notify drivers of potential problems, such as upcoming vehicle maintenance needs or potential traffic backups.
Autonomous vehicles and robotics
Self-driving vehicles and autonomous robots depend on the combination of IoT sensors and big data to operate safely. In a self-driving vehicle, multiple sensor technologies are used, including radar, LIDAR, GPS, and cameras. These IoT components generate a variety of data that the vehicle’s onboard computer uses to create an internal map and chart a course, avoiding obstacles and making split-second decisions. Real-time data analytics is essential in this realm, and the computer can process and learn from experiences over time (machine learning).
Industrial IoT
The industrial IoT (IIoT) applies IoT technology to industries like manufacturing, energy production, and construction. When machines are connected, enterprises can track their activity, progress, and maintenance needs — and ultimately improve operational efficiency. Also called Industry 4.0, IIoT promises to revolutionize manufacturing processes and help organizations take advantage of all types of data.
In a smart factory, pulling together data can be tricky since the typical manufacturing facility encompasses information from a conglomeration of legacy machines, IoT sensors, and manually entered data. IoT gateways equipped with edge analytics can help to streamline data flowing from different sources — often in different formats — and perform initial analyses and data cleansing before sending it to the cloud. At the enterprise cloud level, the factory data undergo more in-depth analyses. The organization’s data science team, equipped with AI and machine learning tools, can then interpret the data and make recommendations for process adjustments to increase efficiency and productivity.
Agriculture
On a smart farm, hundreds, sometimes thousands of IoT sensors can be deployed throughout an agricultural facility — placed far out in a field, inside a remote water tank to monitor usage, or on animals to track grazing. The sensors generate a large body of data on soil conditions, weather patterns, irrigation availability, and other factors. Taken together, the data can provide insights that drive precision agriculture, which takes a focused, individualized approach to cultivation at a particular site. For example, variations between soil conditions in fields on a single farm can be noted and offset with different fertilizing methods.
Retail
Retailers are using IoT devices to track assets, supply chain information, customer behavior, and more. For example, a retailer that sources product materials from multiple locations could equip shipping containers with IoT sensors to monitor movement and ensure that the materials remain in a temperature-controlled environment. Viewed as a whole, the data paints a picture of the supply chain and can be analyzed to reveal insights about how to increase efficiencies or streamline sourcing and assembly.
The future of IoT and big data
As IoT devices become more widely deployed, the amount of data they generate will continue to escalate. To make use of all that information, organizations will need to invest in storage and analytics tools. Edge computing, which processes data in the field or near the edge of the network rather than sending everything back to a cloud server, will likely become more common. Together, big data and IoT will continue to reveal previously unnoticed patterns and present real-time information that helps organizations and individuals make better decisions — or even save lives.
Connectivity with Hologram
To create data sets that are accurate and complete, IoT devices need a dependable source of connectivity. Hologram’s IoT SIM card offers seamless, global coverage for IoT devices with access to LTE/4G/3G/2G technologies. With our Hyper eUICC-enabled SIMs, you’ll gain access to new connectivity partnerships without any additional carrier negotiations, integrations, or hardware swaps.